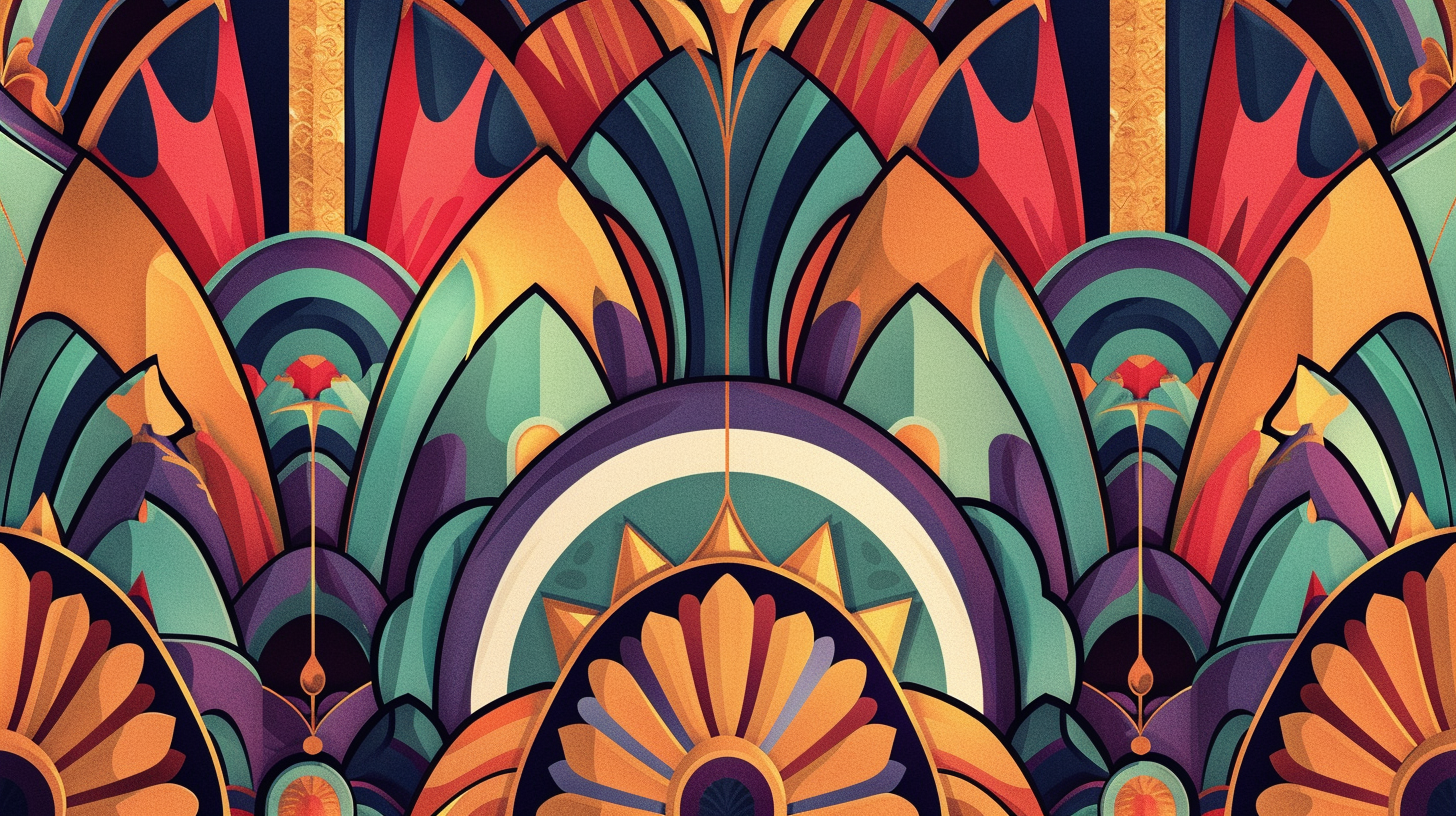
Scaling Out in the Age of AI
Data & Empiricism
Data Analysis for Evidence-Based Results
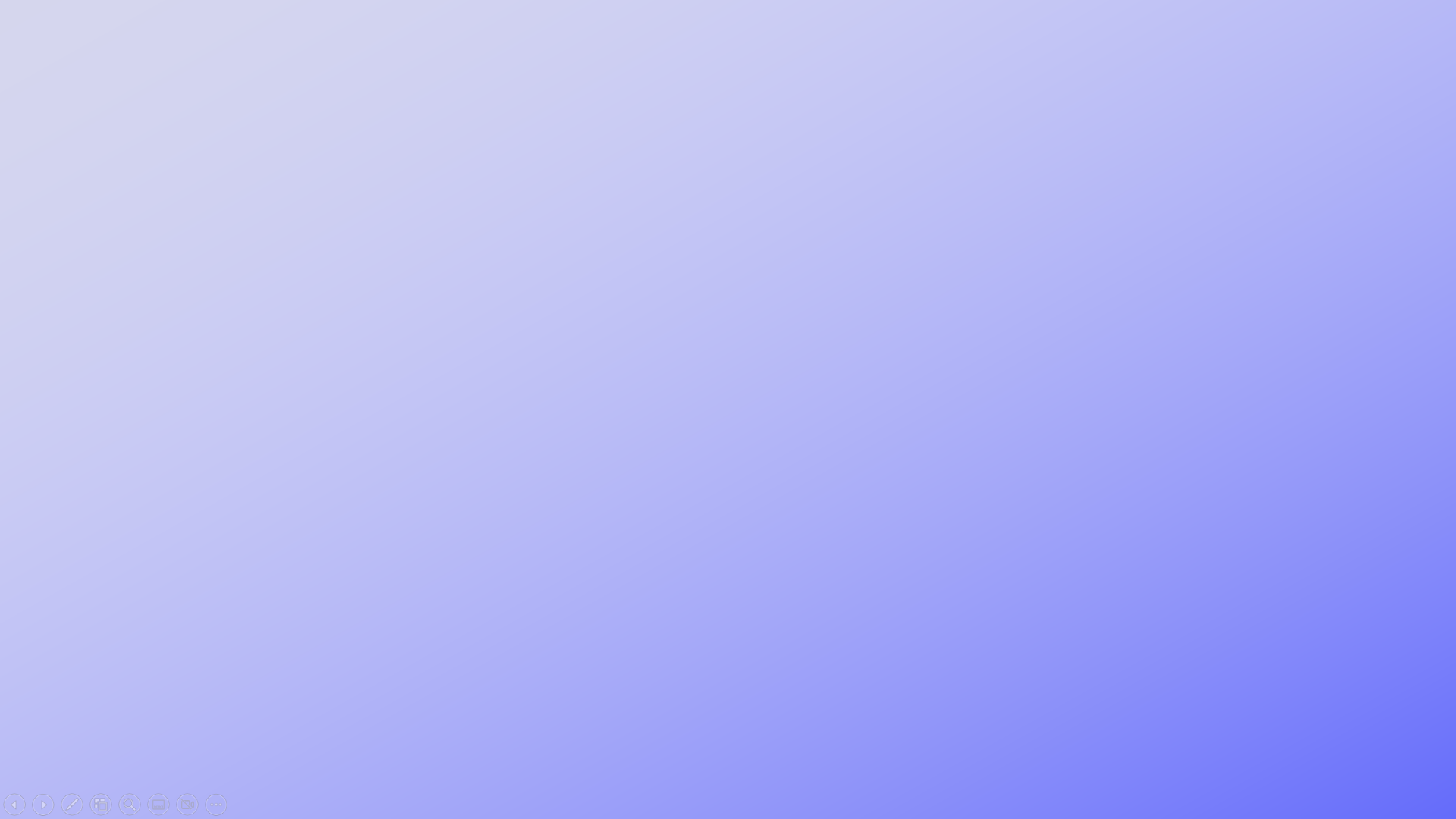
Mini-workshop
(in development)
Format: in-person, online class, or self-paced
Duration: 3 hours
Target Audience: Change Agents and Innovation Champions, Team Leaders and Members in Tech-Driven Fields, Entrepreneurs and Startup Founders, HR and Organizational Development Professionals
Data Analysis for Evidence-Based Results
Are you ready to elevate your data analysis skills and make smarter, data-driven decisions? Our workshop is just what you need!
In this workshop, we'll start with a foundation in data analysis, covering essential techniques for data collection and visualization. We’ll also introduce you to how AI can supercharge your analysis with predictive analytics and machine learning.
Next, we’ll explore the world of metrics and measurement. Learn about the importance of key performance indicators (KPIs), how to identify the ones that matter, and how to use them to measure performance effectively. We’ll also break down different types of metrics—input, process, output, outcome, and impact metrics—so you can evaluate performance from every angle.
Then, we’ll explore experimentation and hypothesis testing. Discover the best methods for formulating and testing hypotheses using data, including statistical testing and experimental design. You’ll also learn how AI can assist in designing, running, and analyzing experiments to uncover valuable insights.
Finally, we’ll focus on goal-setting and evaluation. Using a data-driven approach, you’ll learn frameworks and cycles for setting, monitoring, and evaluating goals, ensuring you stay on track and achieve your objectives.
Learning Objectives:
Master data analysis techniques and enhance them with AI.
Identify and use key performance indicators to measure performance.
Design and analyze experiments to validate hypotheses and set data-driven goals.
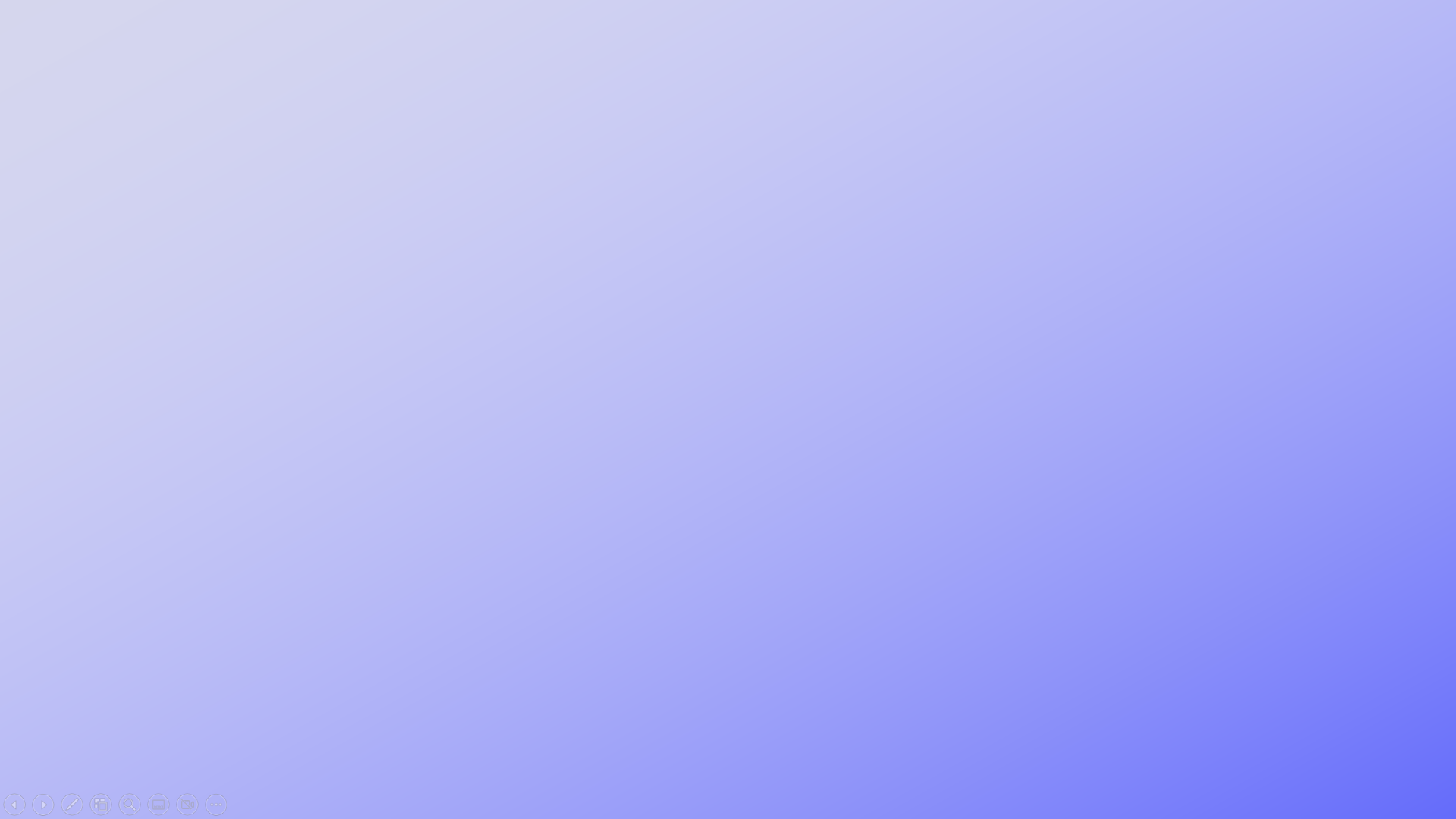
Program
(draft / tentative)
1. Foundations of Data Analysis
Data Analysis
Artificial Intelligence
2. Metrics and Measurement
Key Performance Indicators
Metric categories
3. Experimentation and Hypothesis Testing
Types of Experiments
Validating Hypotheses
Experiments with AI
4. Goal-Setting and Evaluation
Goal-Setting Cycles
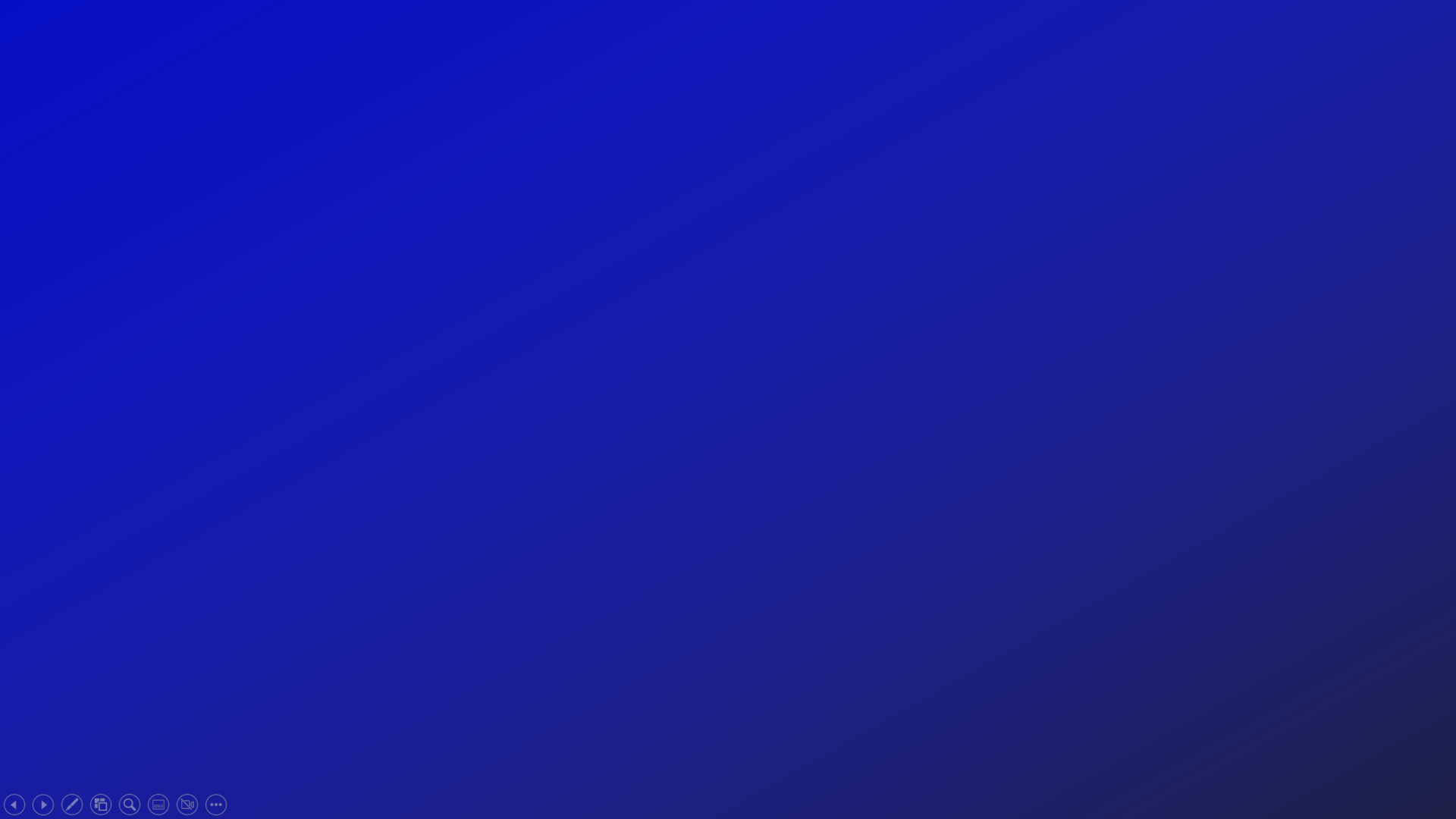
We use our popular unFIX cards in all workshops to boost creative gameplay and engaging discussions. Some examples:
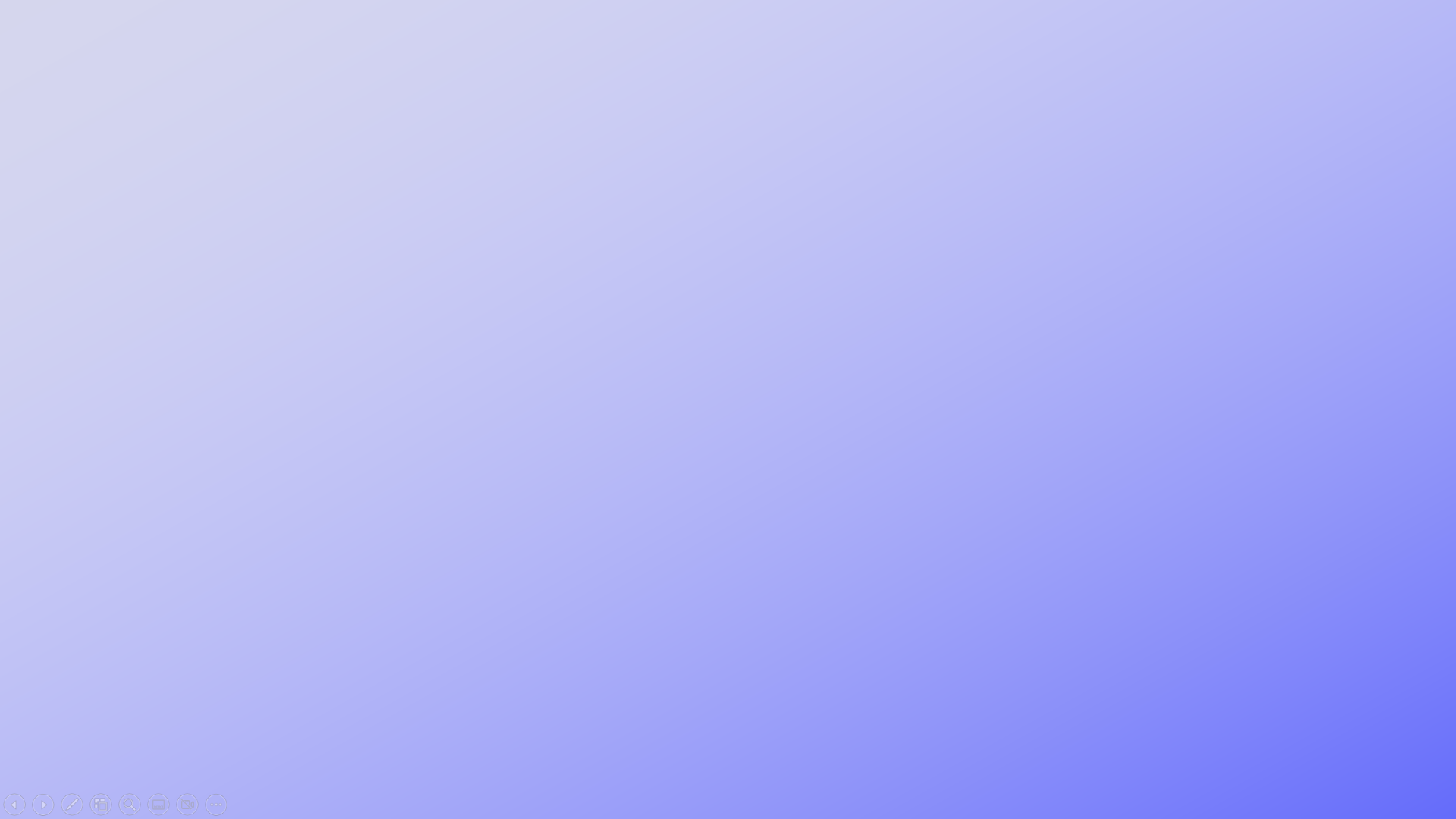
unFIX XL: Scaling Out in the Age of AI (mini-workshops)
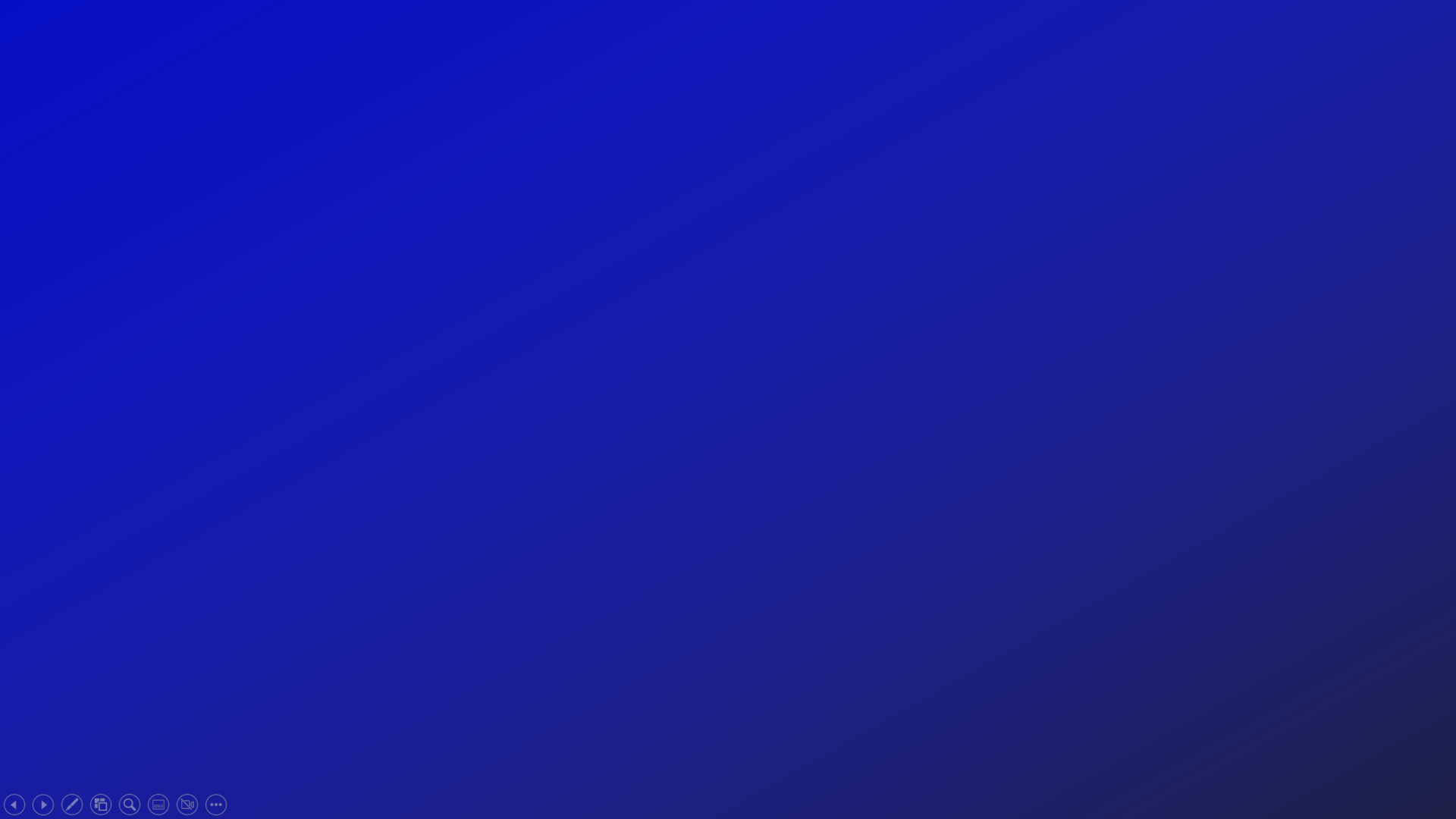
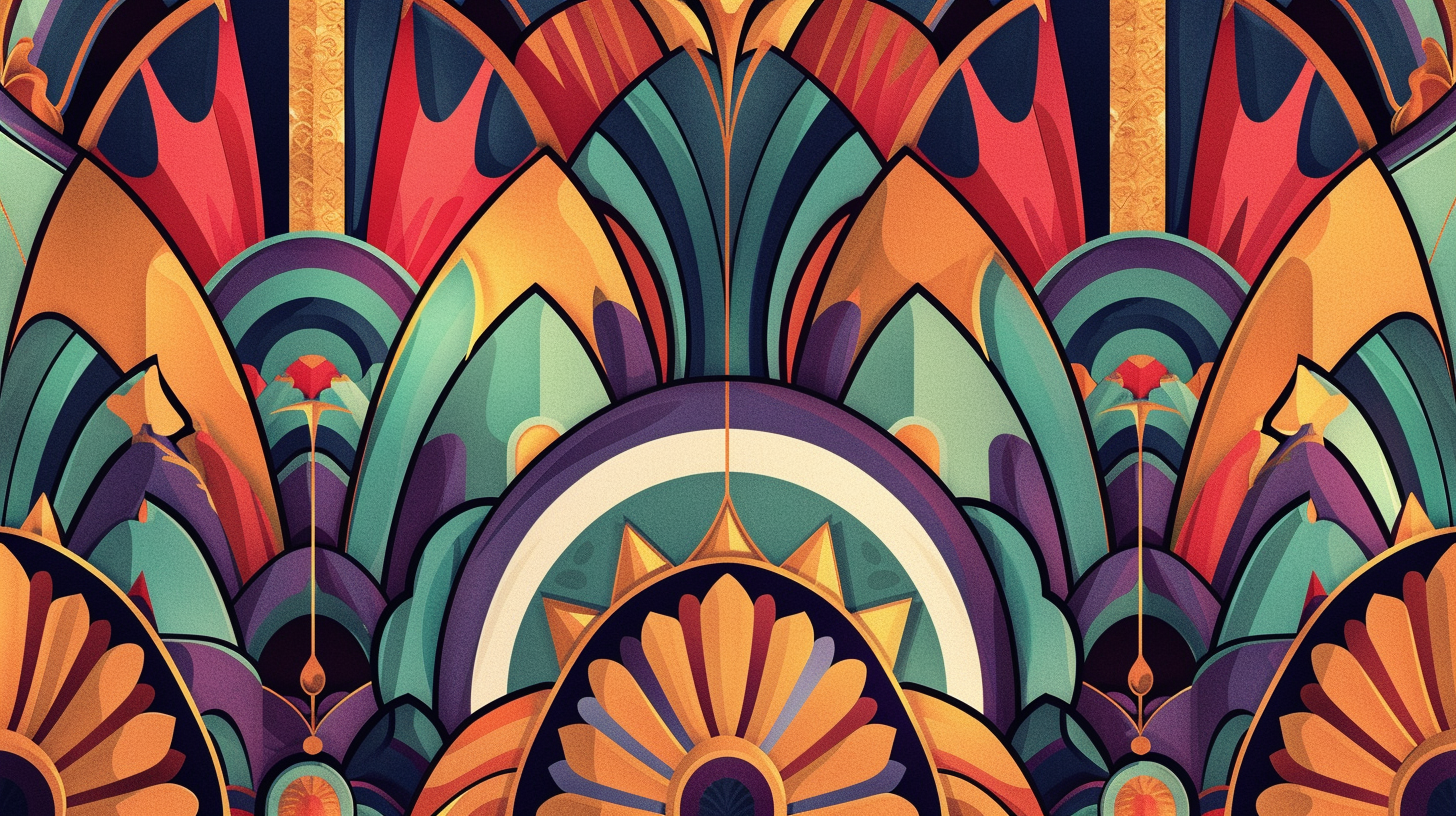